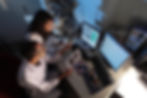
Researchers at the NYU Grossman School of Medicine and Perlmutter Cancer Center have trained a computer to determine which patients with a specific type of skin cancer would respond well to immune checkpoint inhibitor therapy, according to a new study.
In the study, published online ahead of print in the journal Clinical Cancer Research (Nov. 18, 2020), researchers examined patients with metastatic melanoma.
The study’s authors said their findings are important because while immune checkpoint inhibitors have been effective for many patients compared to traditional chemotherapies, half of patients do not respond well.
"Our findings reveal that artificial intelligence is a quick and easy method of predicting how well a melanoma patient will respond to immunotherapy," said Dr. Paul Johannet, in a press release. Dr. Johannet, the study’s first author, is a postdoctoral fellow at NYU Langone Health and its Perlmutter Cancer Center.
Researchers designed their computer program to understand how to get better at a task without being told exactly how to do so. These programs build on mathematical models that enable decision-making based on data examples fed into them, with the program getting more efficient as the amount of training data grows.
For the study, researchers collected 302 images of tumor tissue samples from 121 men and women treated for metastatic melanoma with immune checkpoint inhibitors at NYU Langone hospitals. Then, they divided these slides into 1.2 million portions of pixels, the data that make up digital images. The slides were fed into the computer along with factors such as the severity of the disease, which kind of immunotherapy regimen was used, and whether a patient responded to the treatment.
The study’s authors repeated the process with 40 slides from 30 similar patients at Vanderbilt University to determine whether the results held true from a separate hospital system that used different equipment and sampling techniques.
The researchers note that aside from the computer needed to run the program, all of the materials and information used in the Perlmutter technique are already a standard part of cancer management that most, if not all, clinics use.
"A key advantage of our artificial intelligence program over other approaches such as genetic or blood analysis is that it does not require any special equipment," said Dr. Aristotelis Tsirigos, a co-author of the study. Dr. Tsirigos is the director of applied bioinformatics laboratories and clinical informatics at the Molecular Pathology Lab at NYU Langone.
The study’s authors suggest the AI method is more streamlined than current predictive tools such as analyzing stool samples or genetic information, which promises to reduce treatment costs and speed up patient wait times.
"Even the smallest cancer center could potentially send the data off to a lab with this program for swift analysis," said Dr. Iman Osman, a senior author on the study. Dr. Osman is the Dr. Rudolf L. Baer professor of dermatology at NYU Langone and its Perlmutter Cancer Center.
Dr. Osman added the algorithm will not be ready for clinical use until they can boost the accuracy rate to 90% from 80%. Further testing of the algorithm at additional institutions is also required. The research team now plans to collect more data to better train the computer. Dr. Osman notes that even at its current accuracy, the AI tool can still be used as a screening method to determine which patients across populations would benefit from more in-depth tests prior to treatment.